Solutions > Advanced Tamper Detection
Advanced Tamper Detection
IDmission's cascading Machine Learning models detect fraudulent IDs, deepfakes, digital edits, text changes and photo substitutions.
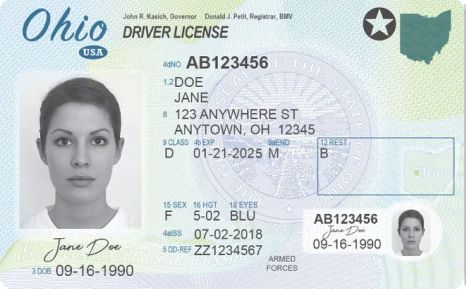
Document Verification
Document Verification Basic requirements for effective Document Verification include:
1. Document detection in complex backgrounds
2. Document extraction, cropping, rotation
3. Detection of primary face, secondary face, MRZ, bar code on document images, front and back
4. Extract data from Machine readable formats (Machine readable zones, bar codes, NFC chips)
5. Accurate OCR of all data
6. Validate Data format and check digits when available
7. Match data extracted from document front with data from machine readable formats
8. Detect presence of national symbols and security features
9. Verify document against issuer databases when available.
Advanced Document Verification Technology addresses numerous new and emerging attack vectors that must be detected and countered.
BPCER - Bona fide Presentation Classification Error Rate
APCER - Attack Presentation Classification Error Rate
Attack Vector | Presentation | Counter Measures | Key Metrics |
---|---|---|---|
Photoshop tamper of photo or text. Physical document not present. | ![]() Physical valid document not present |
Capture technology using mobile or web apps must reject screen images from laptops or mobile devices. | Document Realness Key Metrics: BPCER: < 1% APCER: < 2% |
Tampered printout of a document that was tampered using Photoshop | ![]() Physical valid document not present |
Capture technology using mobile or web apps must reject printouts, photocopies etc. | Document Realness Key Metrics: BPCER: < 1% APCER: < 5% |
Duplicate Faces - Attempted use of a fraudulent photo with multiple legitimate identity documents | ![]() Physical document present, but photo substituted before printing plastic document |
Biometric deduplication will stop the same face from presenting multiple ID documents. | Biometric Deduplication Key Metrics: BPCER: < 0.25% APCER: < 0.0% |
Photo paste up - Photo substitution by pasting a physical photo on an otherwise legitimate document | ![]() Physical document present, but photo physically substituted |
Machine learning models trained to detect physical photo substitution. | Biometric Deduplication Key Metrics: BPCER: < 1% APCER: < 3% |
Text Substitution - by whiteout or ink marker on a physical document | ![]() Physical document present, but text sustituted |
Machine learning models trained to detect physical text tampering on ID documents. | Models are specific to ID type Key Metrics: BPCER: < 1% APCER: < 5% |
AI Deepfake - AI generated fake digital image | ![]() |
DO NOT ALLOW image upload. Cascading series of ML models detects anomalies in AI generated images and prevents upload | Models are specific to ID type Key Metrics: BPCER: < 1% APCER: < 2% |
Injection Attack - Image injection using a virtual camera | ![]() |
DO NOT ALLOW image upload. Capture SDKs from IDmission will disable virtual cameras. |
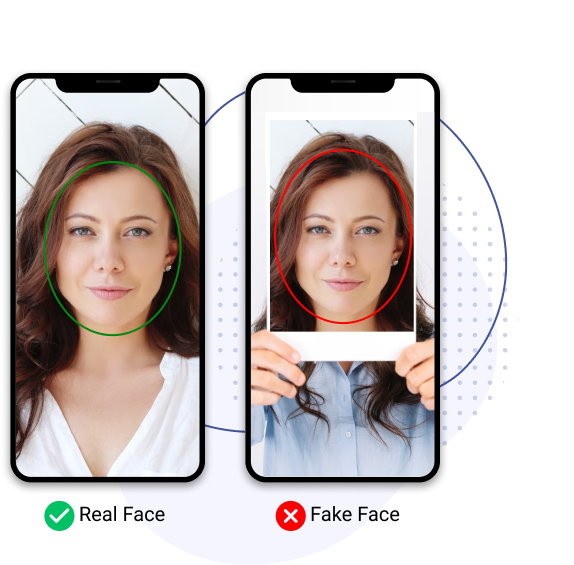
Face Recognition
Basic Requirements for effective biometric facial recognition:
1. Detection of human face
2. Warning if multiple faces in scene
3. Warning to remove COVID masks
4. Warning to remove dark glasses
5. Face looking forward
6. Eyes open
7. Rejection of non-human faces like images of animals
8. Elimination of racial bias
Advanced Face Recognition Technology addresses numerous new and emerging attack vectors that must be detected and countered
Attack Vector | Presentation | Counter Measures | Key Metrics |
---|---|---|---|
Presentation Attack - facsimile of human face (Printout, still image, video, masks, deepfakes, etc.). | ![]() Physical human not present |
Machine learning models trained to detect physical humans and reject any kind of facsimile. ISO 30107-3 Level 1 and Level 2 Passive Presentation Attack Detection certification from iBeta.Passive detection does not require the user to smile, turnhead, blink, or do anything at all | Face Liveness Key Metrics: BPCER: < 1% APCER: < 0% |
Duplication Attack - Same person presenting as different individuals | ![]() |
Instant Biometric deduplication ensures one human can be one customer only. | Biometric
Deduplication Key Metrics: BPCER: < 0.25% APCER: < 0.0% |
Known Fraudster - Known Fraudsters presenting with stolen valid ID documents | ![]() |
Instant matching of each new face against a biometric library of known fraudsters | Negative Biometric
DB Search Key Metrics: BPCER: < 0.25% APCER: < 0.0% |
Facial Deepfake - Deepfakes generated by AI | ![]() |
DO NOT ALLOW image upload. Cascading series of ML models detects anomalies in AI generated images and prevents upload | Proof of Life Key Metrics: BPCER: < 1% APCER: < 0% |
Injection Attack - Image injection using a virtual camera | ![]() |
DO NOT ALLOW image upload. Capture SDKs from IDmission will disable virtual cameras. |
Contact Us Today
We love helping customers solve problems and we are easy to do business with, try us out